Central Limit Theorem (CLT): Definition & Examples
No matter what you want to find out, there is likely a method, calculation, or ratio that can be used. For example, what if you want to forecast the possibility of an event occurring in the future? There’s a method for that.
What if you want to find out more information about a population of people? Yes, there is also a method for this and it’s known as the Central Limit Theorem (CLT). We put together this guide that breaks down exactly what it is, how to use it and its key components. Keep reading to learn more.
Table of Contents
KEY TAKEAWAYS
- CLT is important, as it allows us to use techniques that require normality, even when the underlying data are not necessarily normal.
- The data must be independently distributed. This means that each data point is not affected by the other data points.
- Sample size must be large enough. In general, a sample size of 30 is considered large enough.
- The underlying distribution must be symmetrical. This means that the data are equally likely to be above or below the mean.
What Is the Central Limit Theorem (CLT)?
CLT says that, given a sufficiently large sample size, a distribution of sample means will be approximately normal. This is true regardless of underlying distribution.
In other words, the CLT allows us to make inferences about a population based on a sample. This is true even if we don’t know anything about the underlying distribution.
So, how does this work and what are its uses? Keep reading to find out.
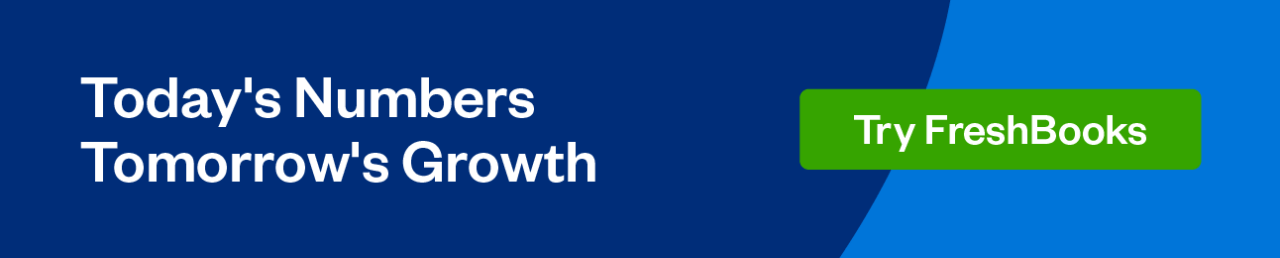
Central Limit Theorem Formula
Central Limit Theorem maintains distribution of sample mean will approach a normal distribution. This is true even as the sample of size gets bigger.
This is true regardless of an underlying population distribution’s shape. So, even if the population is not normally distributed, we can still use the CLT to make inferences about it.
The formula for the Central Limit Theorem is:
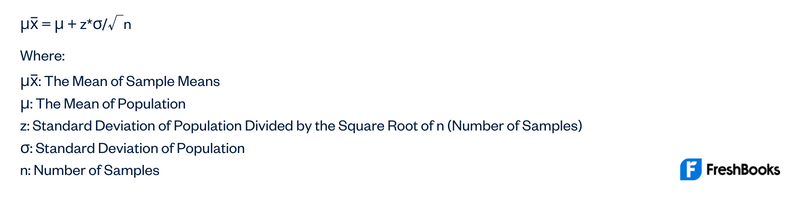
As you can see, the only thing that changes as n gets larger is the z-score. As n approaches infinity, the z-score will approach 0. This means that distribution of sample means will become more and more normal as n gets larger.
The Central Limit Theorem has some important implications. It tells us that we can use normal distribution for inferences about a population even if the underlying distribution is not normal. Second, it gives us a way to calculate the margin of error when we’re taking a sample from a population.
Third, and most importantly, it tells us that the sample mean is an unbiased estimator of population mean. In other words, the sample mean is just as likely to be above the population mean as it is to be below it.
This is a very important result. It means that we can use the sample mean to make inferences about the population without having to worry about bias.
How Does the Central Limit Theorem Work?
The Central Limit Theorem is all about means. In order to understand it, we need to first review some basic concepts.
A population is a group of individuals that we want to make inferences about. A population can be anything from all the people in a city to all the atoms in the universe.
A sample is a subset of the population. We use samples to make inferences about populations.
The mean of population is denoted by μ (mu). It’s simply the sum of all the values in the population divided by the number of values in the population.
The mean of a sample denotes by x̅ (x-bar). It’s simply the sum of all the values in the sample divided by the number of values in the sample.
The standard deviation of population is denoted by σ (sigma). It’s a measure of how spread out the values in the population are.
The standard deviation of a sample is denoted by s. It’s a measure of how spread out the values in the sample are.
Now that we’ve reviewed these concepts, let’s discuss its key components. We will also look at how the Central Limit Theorem works.
Key Components of Central Limit Theorem
There are two key assumptions that must be always met in order for the CLT to hold:
- The sample must be random. This means that each member of the population has an equal chance of selection for the sample.
- The samples must be independent. This means that one sample does not affect the other.
Assuming these two things are true, we can say that:
Suppose sample size (n) increases. Distribution of sample mean (x̄) will become more and more normal, regardless of population distribution’s shape.
In other words, as n gets larger, the distribution of x̄ gets closer and closer to a normal distribution.
This is incredibly useful. We can use the properties of a normal distribution to make inferences about our population. Even if we don’t know anything about its underlying distribution.
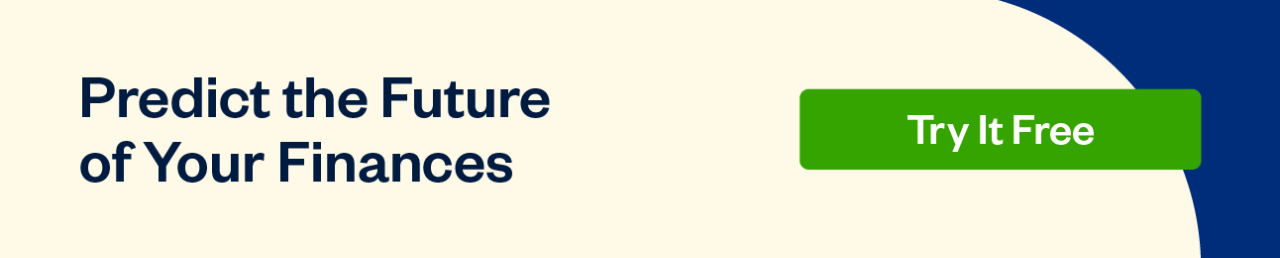
How Is the Central Limit Theorem Used?
The Central Limit Theorem has many important applications in statistics. Some of these applications are:
- Estimating population parameters: We can use the sample mean to estimate the population mean and the sample variance to estimate the population variance.
- Hypothesis testing: CLT can test hypotheses about a population using a variety of test statistics based on the normal distribution.
- Constructing confidence intervals: We can use the CLT to construct confidence intervals for population parameters. This is one of the most common applications of the theorem.
- Modeling data: The CLT is often used in statistical modeling, as many models assume that the data is normally distributed. Even if the underlying data is not normal, we can often transform it in such a way that the resulting data is approximately normal. This allows us to use these models even when the assumptions are not exactly met.
The Central Limit Theorem is a powerful tool that allows us to make inferences about a population based on a sample. It is one of the most important concepts in statistics and has many applications in the real world. If you understand the CLT, you will be well on your way to understanding many other concepts in statistics.
Example of Central Limit Theorem
Let’s say we want to estimate the mean height of all adult women in the United States. We can’t measure the height of every single woman, so we take a random sample of n=100 women and calculate their mean height.
We repeat this process many times and create a distribution of sample means. This distribution is known as sampling mean distribution.
CLT tells us that this sampling distribution will be approximately normal. This is true regardless of population distribution shape.
This means we can use the properties of the normal distribution to make inferences about the population mean based on our sample data.
For example, we can use the sampling distribution to calculate a confidence interval for the population mean.
This interval will give us a range of values that is likely to contain the population mean, based on our sample data.
Summary
CLT is a powerful tool that allows us to use techniques that require normality, even when the underlying data are not necessarily normal. However, it is important to remember that the theorem only holds if the data meet the required conditions. If the data do not meet these conditions, then the results of any statistical techniques used may be inaccurate.
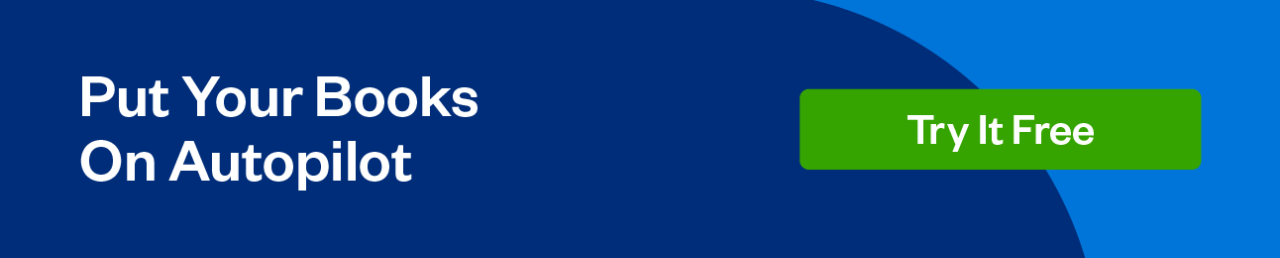
FAQs About Central Limit Theorem
The Central Limit Theorem is important because it allows us to make inferences about a population based on a sample. This is one of the most important concepts in statistics with many applications in the real world.
The three rules of the central limit theorem are:
- Distribution of sample mean will be normal, regardless of population distribution shape.
- A sample’s mean standard deviation is smaller than a population’s standard deviation.
- A sample’s mean standard deviation is equal to a population’s standard deviation when sample size gets divided by its square root.
The central limit theorem has many important applications in statistics. Some of these applications are:
- Estimating population parameters
- Hypothesis testing
- Constructing confidence intervals
Share: