Statistical Significance: Definition & Calculation
In statistics, significance is a measure that determines how likely it is that an observed result is due to random chance. In other words, statistical significance determines one of two things. These are whether or not the results of a study are due to chance or something more meaningful.Ā
Statistics help us make informed decisions about data and avoid being misled by randomness. For example, letās say youāre interested in seeing if eating a certain type of fruit reduces the risk of getting the flu.Ā
Keep reading to learn more about statistical significance and why it matters in your research.
Table of Contents
KEY TAKEAWAYS
- Statistical significance has to do with the results that get generated by a test or experiment. This means that data results are attributable to a specific cause.
- Calculating statistical significance can be difficult to do and is subject to varying degrees of error.
- There are several different types of significance tests that can be used depending on the research needed.
- Some of the common types of significance tests include the T-test, the Chi-squared test, and the one-way ANOVA test.
What Is Statistical Significance?
Statistical significance is the likelihood that an observed result is the result of something. Usually, it’s when the result is more meaningful than random chance. It indicates the strength of an observed result and is typically represented by a p-value.
A p-value is the probability of getting an experimental result or a more extreme result. These could mean a larger sample or smaller sample if the null hypothesis is true. The null hypothesis assumes that nothing happened. And that there was no significant difference between the experimental group and the control group.
While significance measures how likely it is that your results are due to chance, it does not tell you if the results are good or bad. For example, a significant difference in the results of two groups can indicate one group has a much higher incidence of disease than the other.
The significance merely informs you that there is a difference between the two groups and how likely it is that this difference is due to chance.
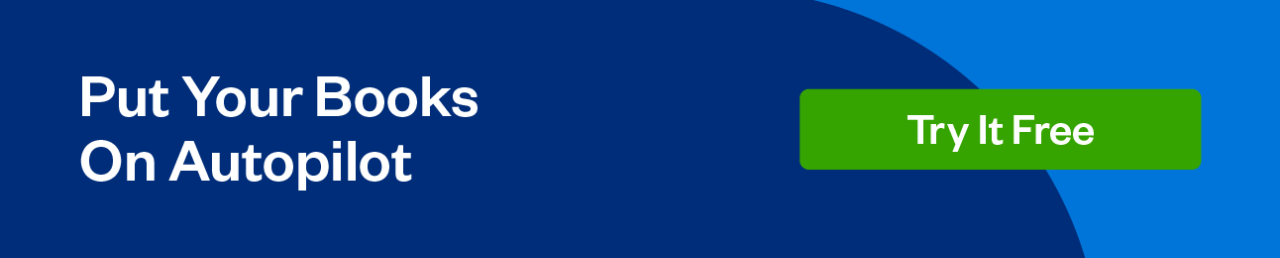
Types of Statistical Significance Tests
There are many types of statistical significance tests and each has a different level of significance. The most common tests are the T-test, the chi-squared test, and the Z-test. Letās take a look at each one.
T-Test
The t-test is used to determine whether there is a significant difference between two means. Specifically, it compares the mean of a control group to the mean of an experimental group. This helps determine if there is a significant difference between the two groups.
Moreover, the t-test determines if the difference between the means is beyond what you would expect from sampling error.
One-Way ANOVA
ANOVA test is used to determine whether there are significant differences between three or more means. It determines whether there is a difference between each mean and the level of significance is determined by the F-test.
Chi-Squared Test
This test is used to determine whether two categorical variables show a significant relationship. A categorical variable is one that can only take on certain values. For example, blood type and gender are both categorical variables.
Z-Test
This test is used to determine whether a populationās mean and standard deviation differ from another. You use Z-tests when you have a sample mean, a sample standard deviation, and a population mean you wish to compare against.
How Is Statistical Significance Used?
If you run a t-test and get a p-value of less than 0.05, then your results are statistically significant. This means you have a 5% chance of obtaining this result if there is no difference between the two groups.
If you want to be more specific, you can look up your p-value to see how likely it is that youāre observing a false positive.
False positives occur when your results do not provide an accurate conclusion. For example, let’s say you conduct a test for the presence of a certain chemical in water. You get a positive result (i.e. the chemical is present in the water) but this result is actually false.
A false positive shows that there is no practical significance between the results of your experiment and the control group. If you have a false positive, it is not a good idea to take action based on the results of your experiment.
Researchers can avoid false positives when looking at results of their study. They then use the p-value to determine if their results are statistically significant.
It is important to note that a statistically significant result does not mean that there is a causal relationship between the groups. It only means that the groups are different and that this difference is unlikely to have been caused by chance.
To determine if there’s a causal relationship between groups, researchers conduct extra research. This could be exploring the relationship between the two groups.
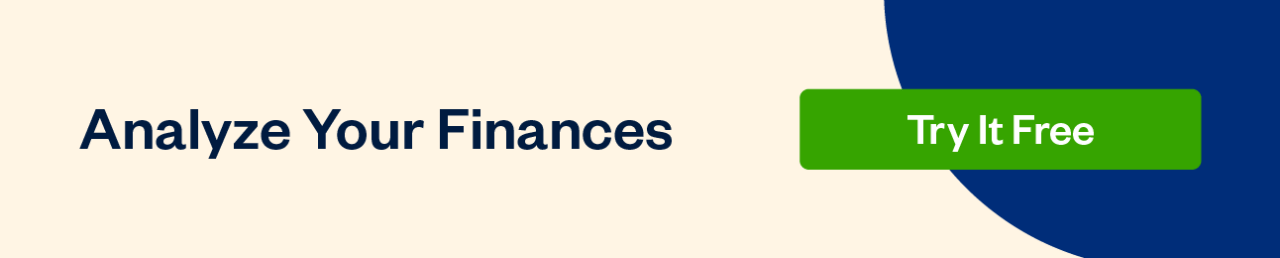
Why Is Statistical Significance Important for Business?
A data scientist must determine the level of significance when conducting a statistical analysis. If a result is statistically significant, then itās more likely that the outcome is due to something more meaningful than chance.
This means the results of your statistical analysis are reliable and can be applied to real-world problems. It’s important for businesses since it enables data scientists to apply the results of their analysis.
If a statistical result is not significant, it’s likely the outcome is due to chance. This means it’s not a meaningful relationship between the experimental group and control group.
A statistically significant result gives confidence that the results are real and not random by chance.
This means that the results of your analysis have a better chance of being applicable to your business and can be used to inform future decisions.
Examples of Statistical Significance
The following scenarios showcase how you can use statistical significance to determine if a result is meaningful or if it is just by chance.
Imagine you have a hypothesis that eating 10 pieces of fruit per day reduces the risk of getting sick. You test this hypothesis by running an experiment with 100 people, dividing them into two groups.
An experimental group that eats 10 pieces of fruit a day and a control group that does not eat any fruit at all. If there are few people in both groups that get sick at the end of the experiment, your results are not statistically significant.
Another example is if you have a hypothesis testing that eating 10 pieces of fruit per day reduces the risk of getting sick. You run an experiment with 100 people dividing them into two groups. Eating 10 pieces of fruit per day does not seem to reduce the risk of getting sick.
In both of these scenarios, you did not obtain statistically significant results. However, this does not mean your hypothesis is incorrect. It just means that there was not enough evidence to prove the hypothesis one way or another.
You can also use statistical significance as a measure for attention deficit, a physiological measure, and more. The goal is to use statistical power to support an alternative hypothesis.
Whatever types of significance tests you use, you can be sure to gain valuable insights from your data. You’ll have a better degree of confidence in your statistical tests.
Summary
A hypothesis is a theory that has not been proven. When conducting an experiment, researchers will form a hypothesis and then test it using statistical significance.
If a statistical result is statistically significant, it means that it is likely not the result of chance and is a more accurate representation of the truth.
Statistical significance is important for businesses because it allows data scientists to confidently apply the results of their analysis to real-world problems.
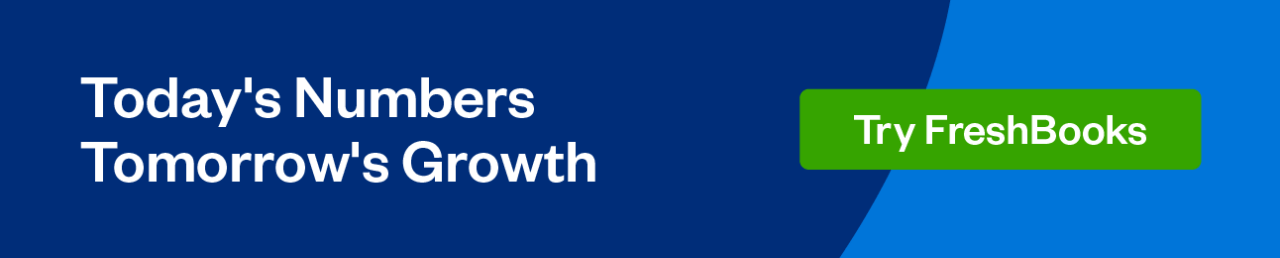
FAQs About Statistical Significance
Statistical significance measures how unlikely it is that an experimental result is due to chance. No matter what the significance level is, a significant result is one that is unlikely to be due to chance.
The significance level is the probability that an experimental result is due to chance. For example, a significance level of 0.05 means that thereās a 5% chance that the result is due to chance. The lower your significance level is, the more confident you can be that your result isnāt due to chance.
A 5% or less p-value of is generally considered to be statistically significant.
Both are measures of how unlikely it is that an experimental result is due to chance. While significance measures the probability that a result is due to chance at a specific level, confidence level measures the probability that a result is due to chance at any level.
Share: